Imagine a computer system that was able to monitor and evaluate the critical data for your organisation – making sure it is complete and accurate – in real time
AI and machine learning are getting a lot of attention these days. It is being, with varying (and sometimes amusing) degrees of success to write articles, speeches and even computer code. But these fast-evolving technologies also offer impressive opportunities for things that can – and should – streamline and enhance the accuracy, completeness and timeliness of data collection.
One of the complications of ESG data collection is the myriad of data sources that need to be combined, organized, error-checked and calculated to arrive at comparable, reliable and decision-useful information. If you think about Greenhouse Gas emissions – even something as straightforward as using electricity bills to calculate the emissions associated with that energy use is complicated by needing 12 months of data, for each location, taking into consideration the energy mix where the power is drawn from. Making sure that the data needed is complete and covers the entire reporting period takes a lot of time and effort. But AI promises – yet to be validated – to use the power of computing to ‘figure out’ if something doesn’t look right (i.e., missing a portion of the data).
ESG professionals, disclosure committees and even auditors rarely look at all of the data. They validate the methodology, use a representative sample of the reporting to see if it gives them ‘reasonable’ confidence in the information.
These technological advances have the potential to change all that. Imagine a computer system that was able to monitor and evaluate the critical data for your organization – making sure it is complete and accurate in real time – rather than every year. It is a lot easier for a system to determine – there are fewer bills/less data sources than expected. It might even be able to flag where the data is missing.
Why does this matter? Because the old saying ‘that which is measured gets managed’ does not go far enough. That which is measured well gets managed well. But that which is measured poorly is often mismanaged. And AI/machine learning can be a valuable tool to make sure that you are measuring and managing things well – from financial performance, to safety, to environmental impacts, and more.
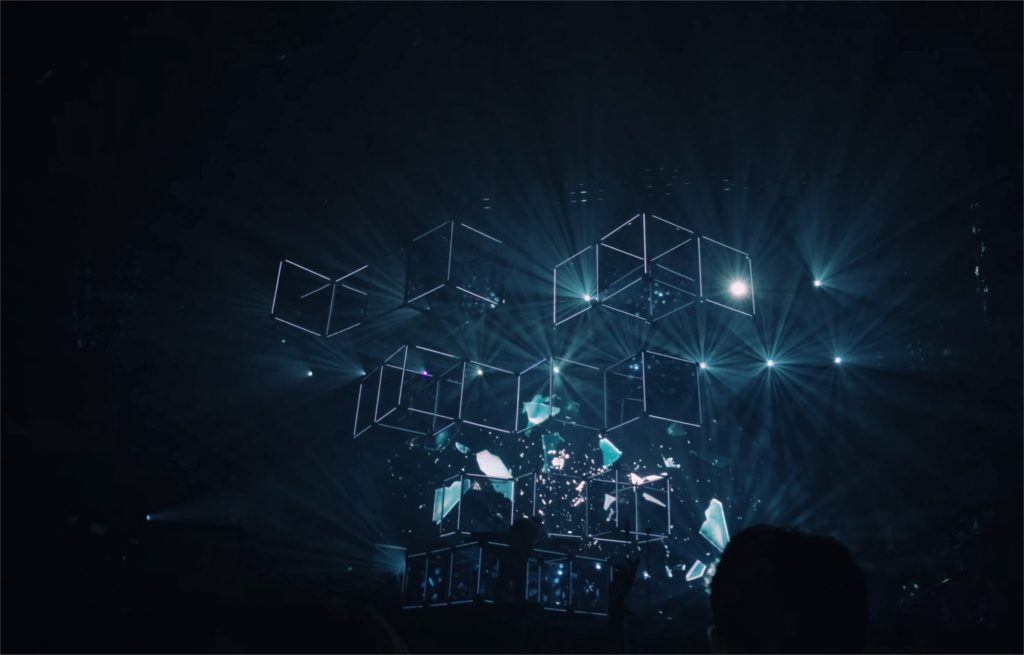
That which is measured well gets managed well. But that which is measured poorly is often mismanaged.
What would it do to management efficiency if the data were to be processed and scrutinized in this way? Likely increased confidence in the basis for decision-making. And the same holds true for other stakeholders – like investors – who likely will be VERY interested in companies that are ‘error checking’ their data in real time and using that to make more informed, faster, and more nimble management decisions.
Thanks to John Friedman who inspired us to share thoughts on this subject.